Predicting Employee Turnover
The primary goal was to help Salifort Motors understand the key reasons why employees may leave the company. Addressing this issue can help organizations retain talent and reduce turnover costs.
MACHINE LEARNING
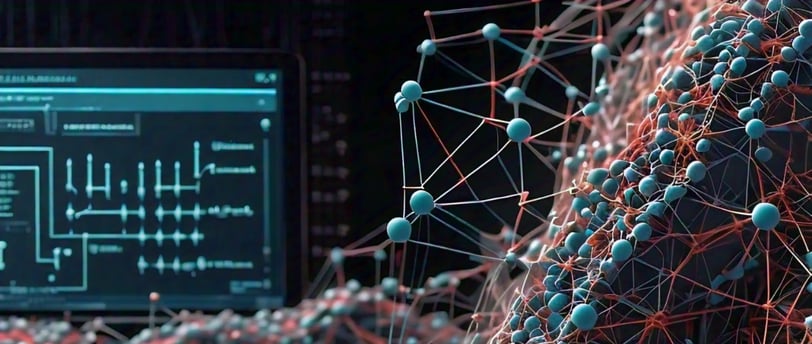
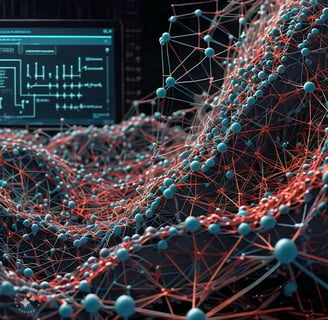
Project Overview:
In this project, I worked on predicting employee turnover using decision tree and random forest models. By leveraging a dataset of employee characteristics, I aimed to identify the most important factors influencing employee retention. The final model provided insights that can assist HR in improving retention efforts.
Business Context:
The primary goal was to help Salifort Motors understand the key reasons why employees may leave the company. Addressing this issue can help organizations retain talent and reduce turnover costs.
Data Summary:
The project used a dataset containing features like performance evaluations, tenure, salary, and workload. By analyzing this data, I gained an understanding of the underlying factors affecting employee retention.
Modeling:
I applied decision trees and random forest algorithms to predict employee turnover. The random forest model slightly outperformed the decision tree, pinpointing important features such as performance evaluations, the number of projects, and tenure.
Conclusion:
The analysis revealed that high workloads, overwork, and performance evaluation scores play a crucial role in employees' decisions to leave. These insights can be used by HR teams to refine policies and improve overall employee satisfaction.
Location
Karachi, Pakistan
Contacts
+92 336-2349646
ammarullahkhan5@gmail.com
Expertise
Transforming data into impactful stories for my clients